Abstract
Function annotation is a challenging problem in Computational Biology. Relying on evolutionary relationships is often suboptimal for function assignment. In a collaborative work, we have extensively tested various deep learning-based methods (CNNs and LMs) on full proteomes to assess their performance at the organism level. We found that transformer-based protein language models are more precise and informative than other methods for all the species tested and across the three gene ontologies studied. They also better recover functional information from transcriptomic experiments. We applied the best methods to annotate 1,000 species from the animal phyla, and have produced the FANTASIA pipeline to finding that functional recovery can effectively address experimental hypotheses.
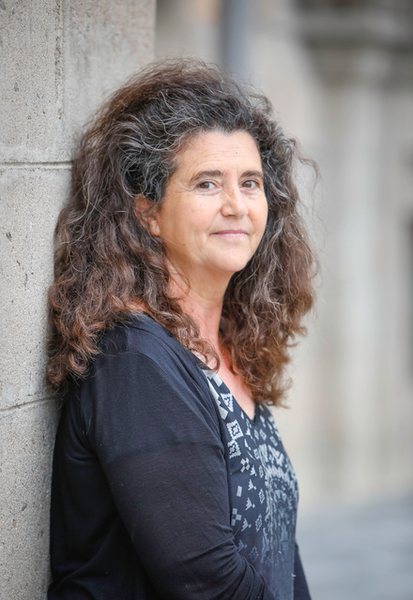