Objectives
Abstract:
The drug discovery process is challenging, time-consuming, and extremely expensive. Drug repurposing is a strategy for identifying new uses for existing drugs, aiming to simplify the process. While machine learning approaches have shown promise, they often need more mechanistic understanding, which is necessary for robust drug target identification and repurposing strategies. Mechanistic models provide crucial insights into the underlying biological mechanisms, complementing machine learning techniques. However, inferring mechanistic signaling networks from omics data poses challenges due to non-identifiability, resulting in multiple valid solutions consistent with the data.
In this talk, we will explore the applications of neural networks and multilayer biological networks for drug repurposing opportunities and network inference problems applied to signaling pathways.
In the first part, we present a novel machine learning and network-based workflow to identify drug targets for cystinosis, the most common cause of inherited tubular dysfunction and kidney disease in children and currently lacks effective therapies. This approach allows us to recapitulate the disease mechanisms in the context of renal tubular physiology and identify candidate drug targets for further validation using a cross-species workflow and disease-relevant screening technologies.
In the second part of the presentation, we shift our focus toward quantifying signaling model diversity through solver-agnostic solution sampling with CORNETO, which is an ongoing effort that aims to unify network inference problems via constrained optimization.
Mechanistic signaling networks can be inferred from omics data and prior knowledge using combinatorial optimization and mathematical solvers to find the optimal network. However, this problem is in general, non-identifiable, and several solutions may be equally valid. Ignoring the existence of these alternative solutions leads to an incomplete picture of the hypothesis space of consistent mechanistic signaling networks. To alleviate this issue, we implemented an algorithm to explore the space of alternative solutions and to conduct sensitivity analysis on the optimal solution.
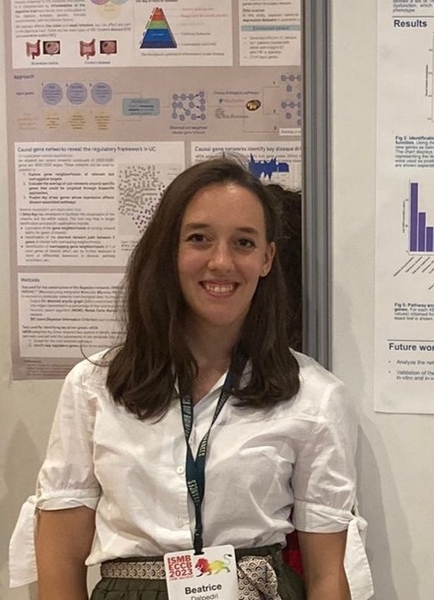
Beatrice Dalpedre obtained her master's degree in Biostatistics at the University of Milan-Bicocca. She first worked as a biostatistician and data scientist in the Transplant Coordination Department of the Policlino in Milan and later at the Bruno Kessler Foundation (Trento, Italy). Currently, she is finishing her Ph.D. in Computational Biology at the University of Trento-COSBI. Her main work focuses on applying deep learning and network methodologies for drug repurposing in rare diseases. She has recently concluded a visiting research period at the Saez-Rodriguez Laboratory at Heidelberg University where she worked on sensitivity analysis of optimized signaling pathways models.
Speakers
Speaker: Beatrice Dalpedri. Ph.D. in Computational Biology at the University of Trento-COSBI.
Host: Alfonso Valencia, Computational Biology -Life Sciences Group, BSC.